The Best Strategy for AI Deployment
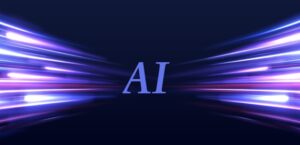
(Mirotvoric/Shutterstock)
With AI failure historically reported to be around 85%, there is every reason to step back and consider that most approaches and most vendors supplying AI solutions aren’t working. This same kind of failure rate has been problematic for most every technology wave I’ve covered, starting with the Client/Server wave which was, for the first decade, a huge failure that no one wanted to talk about.
Back when I was a competitive analyst and a member of SCIP (Society of Competitive Intelligence Professionals), I took a class that has always stuck with me. The professor drew a typical X/Y chart with direction on the vertical axis and speed on the horizontal access. He pointed out that the problem with most companies is they operate in the lower right quadrant, which means they focus on speed first, when they should be in the upper left quadrant focused on direction first. His conclusion seemed obvious. If you focus on speed first and not direction, you are only going in the wrong direction more quickly, which could be a terrible thing.
Let’s talk about the strategic approach to AI deployment.
Setting Direction
One of the problems with a recent technology wave is that everyone seems to look at everyone else for direction not realizing that most have no idea what the direction should be because they have yet to learn the capabilities, shortcomings and advantages of the recent technology. As a result, they tend to run ever faster in a direction that will be unsuccessful at a sadly increasing rate.
The problem right now is that while there are two companies, NVIDIA and IBM, that seem to understand the power and limitations of AI because they’ve been at it the longest, they are technology suppliers and not held up as examples of technology use excellence. NVIDIA, more so than IBM, is a heavy user of the technology, but its business is hugely different from its customers’, so its best practices, which have clearly proven to be successful, aren’t being widely used as global examples of excellence.
Additionally, this early on in a massive technological disruption, the direction of the technology isn’t truly set because there simply isn’t enough adoption yet. The technology itself is undergoing massive change as AI is applied to develop future AI advancements at an increasingly blistering pace that shows no signs of slowing.
This suggests that there needs to be three well maintained internal lists that are fact, not aspirational, based. One list is a priority of what the company needs AI to do coupled with the reason for the ranking. For instance, a company experiencing mass employee shortages may have AI employee replacement as a top priority so that it can more efficiently and effectively operate. The second list, which is equally dynamic, is what AI can economically (the economic part is important) do based on what others are using it successfully for. The final list is where the two lists converge and provide a list of things the company needs to do ranked by both company priority and tool capability.
Focus on Flexibility
Any resulting plan should be designed to be highly flexible because both the company’s needs and AI capabilities are in flux and likely to continue fluxing during this time of massive change.
This means that decision makers have to be open to and looking for changes that will either force a plan update or change wrapped with the concept of “sunk cost.” In other words, decisions to pivot shouldn’t be based on the money and effort already made, but on the money and effort that will need to be made to adapt to the new goal. If you find you are going in the wrong direction, you don’t want to continue in that direction just because you’ve spent a lot of money getting to the wrong place.
Flexibility will be key to success because, just as no military plan survives first contact with the enemy, no IT plan will survive intact once implementation starts, particularly when the underlying technology is changing as rapidly as this one is.
Therefore, assuring flexibility and the ability to hard pivot is always on the table are both critical aspects of a successful AI deployment strategy.
Wrapping It Up
It is ironic that knowledge-based technology is being badly hurt by the lack of knowledge and experience in that same technology, but that is where we are. I could argue that, as a result, the best initial focus for an AI solution would be to help with future AI project decisions so that the tool itself is used to better assure its successful application.
We clearly aren’t there right now, so my best advice is to assure your decisions are backed by research into both what you need done and the actual capabilities of the tool and that they remain flexible because the foundations for your decision will remain in flux for the foreseeable future.
About the author: As President and Principal Analyst of the Enderle Group, Rob Enderle provides regional and global companies with guidance in how to create credible dialogue with the market, target customer needs, create new business opportunities, anticipate technology changes, select vendors and products, and practice zero dollar marketing. For over 20 years Rob has worked for and with companies like Microsoft, HP, IBM, Dell, Toshiba, Gateway, Sony, USAA, Texas Instruments, AMD, Intel, Credit Suisse First Boston, ROLM, and Siemens.
Related Items:
How HP Was Able to Leapfrog Other PC/Workstation OEMs to Launch its AI Solution
Why Digital Transformations Failed and AI Implementations Are Likely To
2024 and the Danger of the Logarithmic AI Wave